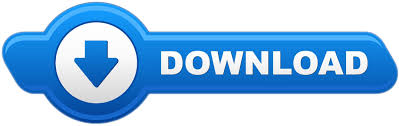
However, training these kinds of networks is notoriously difficult, since the error back-propagation mechanism commonly used in conventional neural networks cannot be directly transferred to SNNs due to the non-differentiability at spike times. Hence, mobile robots will be able to manage their weaknesses of carrying limited computing resources and power supply based on SNN-based controllers. Overall, SNNs have great potential to offer an accurate and efficient way to model the principles underlying neural structures devoted to locomotion control in living creatures. Yet, the human brain only needs remarkably low power consumption, which is around 20 W of power ( Drubach, 2000).

Furthermore, in terms of energy efficiency, maintaining the sufficient functions of the nervous system to perform various tasks requires a continuous energy supply ( Schoettle and Sivak, 2014). For example, experiments have demonstrated that the visual pattern analysis and pattern classification can be carried out by humans in just 100 ms, in spite of the fact that it involves a minimum of 10 synaptic stages from the retina to the temporal lobe ( Thorpe et al., 2001). Since SNNs are able to transmit and receive large volumes of data encoded by the relative timing of only a few spikes, this also leads to the possibility of very fast and energy-efficient computing. This eliminates the need for an averaging time window and allows for processing information in continuous time, greatly reducing response latencies ( Frémaux et al., 2013). An advantage of this form of information processing is the possibility of not only encoding spatial information like traditional ANNs do, but also adding temporal information in the form of the precise timing of spikes ( Maass, 1997). Different from this, SNNs process information in the form of pulses or spikes, which is much more similar to the natural nervous system, and therefore, more biologically realistic and plausible. Since traditional ANN computing units process signals in the form of continuous activation functions, they can be interpreted as their average pulse frequencies over a time window.

We demonstrate the performance and effectiveness of our trained SNNs to achieve target reaching tasks in different unknown scenarios.ĭespite the success of traditional artificial neural networks (ANNs) in learning complex non-linear functions, the interest of spiking neural networks (SNNs) is steadily increasing due to the fact that SNNs offer many fundamental and inherent advantages over traditional ANNs, such as biological plausibility ( Maass, 1997), rapid information processing ( Thorpe et al., 2001 Wysoski et al., 2010), and energy efficiency ( Drubach, 2000 Cassidy et al., 2014). Together they make up a target-reaching controller, which is used to control a simulated mobile robot to reach a target area while avoiding obstacles in its path. We use the supervised reward-modulated Spike-Timing-Dependent-Plasticity learning rule to train two different SNN-based sub-controllers to replicate a desired obstacle avoiding and goal approaching behavior, provided by pre-generated datasets. This paper addresses the problem by introducing an end-to-end learning approach of spiking neural networks constructed with one hidden layer and reward-modulated Spike-Timing-Dependent Plasticity (R-STDP) synapses in an all-to-all fashion. This results in neglecting one of the biggest advantages of ANNs, i.e., being general-purpose and easy-to-use due to their simple network architecture, which usually consists of an input layer, one or multiple hidden layers and an output layer. Although SNNs have been used to solve a variety of control tasks using the Spike-Timing-Dependent Plasticity (STDP) learning rule, existing solutions usually involve hard-coded network architectures solving specific tasks rather than solving different kinds of tasks generally. Spiking neural networks (SNNs) offer many advantages over traditional artificial neural networks (ANNs) such as biological plausibility, fast information processing, and energy efficiency.
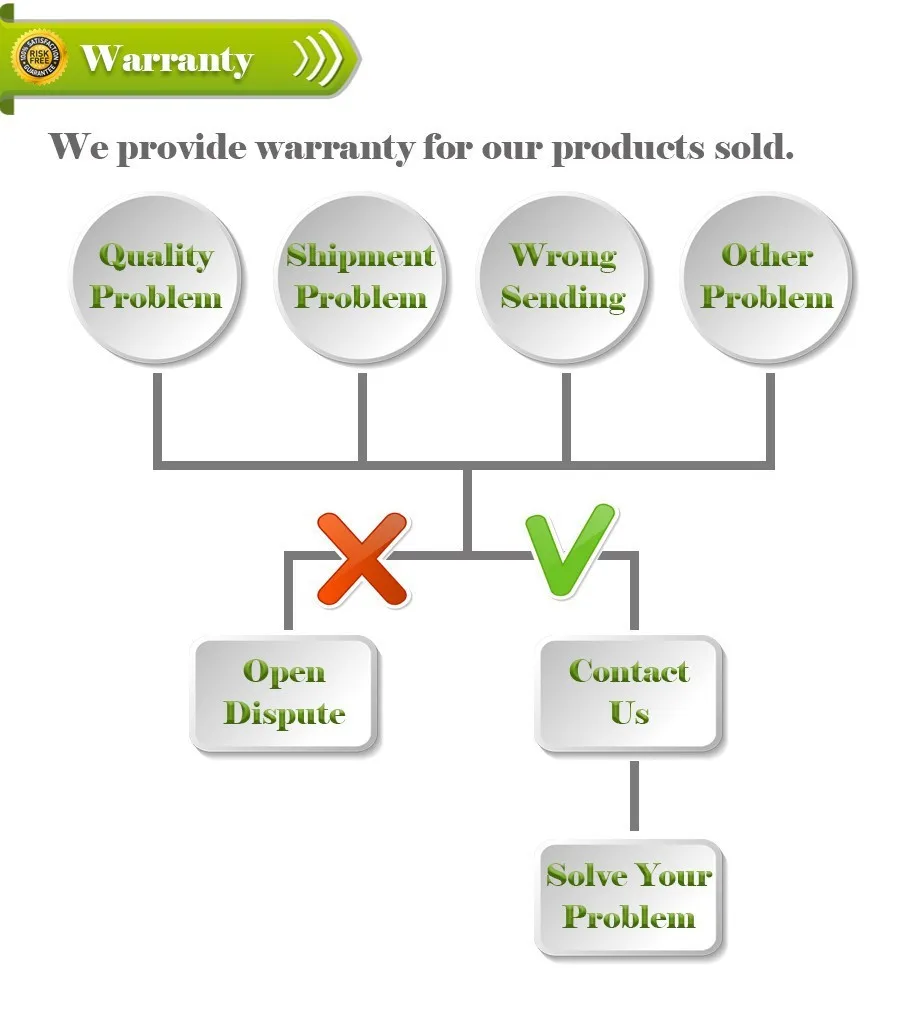
Zhenshan Bing 1, Ivan Baumann 1, Zhuangyi Jiang 1, Kai Huang 2,3, Caixia Cai 1 * and Alois Knoll 1
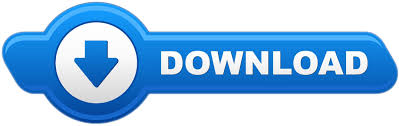